On the Interaction between Software Engineers and Data Scientists when building Machine Learning-Enabled Systems
In recent years, Machine Learning (ML) components have been increasingly integrated into the core systems of organizations across diverse sectors, including e-commerce, healthcare, and finance. Engineering such systems presents various challenges from both a theoretical and practical perspective. One of the key challenges is the effective interaction between actors with different backgrounds who need to work closely together, such as software engineers and data scientists. In this paper, we present an exploratory case study that aims to understand the current interaction and collaboration dynamics between these two roles in ML projects. We conducted semi-structured interviews with four practitioners with experience in software engineering and data science of a large ML-enabled system project and analyzed the data using reflexive thematic analysis. Our findings reveal several challenges that can hinder collaboration between software engineers and data scientists, including differences in technical expertise, unclear definitions of each role’s duties, and the lack of documents that support the specification of the ML-enabled system. We also indicate potential solutions to address these challenges, such as fostering a collaborative culture, encouraging team communication, and producing concise system documentation. This study contributes to understanding the complex dynamics between software engineers and data scientists in ML projects and provides insights for improving collaboration and communication in this context. We encourage future studies investigating this interaction in other projects.
Authors: Gabriel Busquim, Hugo Villamizar, Maria Julia Lima and Marcos Kalinowski
Vortragssprache: Englisch
Level: Fortgeschrittene
Zielgruppe:
Unternehmen:
Pontifical Catholic University of Rio de Janeiro
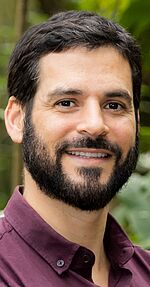
Prof. Dr. Marcos Kalinowski